Fraud is a growing concern for enterprises, banks, and customers. As the dimension of digital transactions and online activities grows, so invariably does the potential for fraud.
The old fraud detection methodologies, relying heavily on rule-based systems and manual work, have become too slow to keep pace with technologically advanced fraudsters.
Therefore, AI is becoming a force to reckon with in bringing real-time, adaptable, and very effective fraud detection and prevention systems.
AI fraud detection techniques are based on ML, deep learning, and data analytics, resulting in a much higher detection precision of every suspected fraudulent activity under consideration.
The article discusses the operation of AI fraud detection, its advantages, challenges, and important strategies to utilize AI for fraud prevention.
What Is AI Fraud Detection?

AI fraud detection implies using artificial intelligence and machine learning techniques to detect, analyze, and prevent fraudulent activities in real time.
As large volumes of data are processed, AI detects the anomalies and patterns of fraudulent activities and responds in real time to prevent monetary gain.
AI fraud detection is applied in almost any domain, including banks, e-commerce, health care, insurance, and others, to protect sensitive data and monetary transactions.
How Are AI Fraud Detection Systems Working?

AI fraud detection is a series of multi-step processes that include large-scale data collection, pattern recognition, learning, and real-time action. The main steps include;
1. Data Collection
AI fraud detection tools gather and analyze almost unlimited data across multiple sources, such as transaction history, logging points, IP addresses, device fingerprints, behavioral biometrics, and financial data.
The larger the data sets, the more properly AI can tell one real transaction from another alleged fraudulent transaction.
2. Feature Engineering
It is the activity of selecting raw data. This process converts useful indicators of fraud. AI models pick out important features like anomalous transaction values, a sequence of rapid consecutive transactions, or anomalous geographic patterns that can indicate fraudulent behavior.
3. Model Training
AI models are trained on historical fraud data. Supervised learning algorithms apply labeled data, where previous transactions are labeled as fraudulent and legitimate, creating a map based on learned patterns.
Meanwhile, the unsupervised approach contributes to pattern recognition in unknown fraud by detecting anomalies from new data.
4. Continuous Learning
One of AI fraud detection’s strongest selling points is its ability to learn and develop with time. By continually observing new fraud events and fine-tuning its algorithms.
AI has been sharpening its detection powers and learning to stay one step ahead in the battle with increasingly complicated fraud techniques.
5. Alerting And Reporting
AI fraud detection systems send alerts almost instantly whenever suspicious activity is detected. Immediately taking action, such as blocking transactions, observing accounts, or notifying users, is possible.
Moreover, organizations that benefit from AI-powered dashboards can analyze current fraud trends and keep security proofing an active endeavor.
Benefits Of AI Fraud Detection
Whenever we talk about any technology, there are definite benefits attached to it, and it is very important to understand these benefits to be able to use them in their entirety.
A. Real-Time Detection And Prevention
With the help of AI, organizations can now detect fraudulent activities in real-time, thus minimizing financial theft losses and enabling entry into unauthorized spaces.
Real-time processing enables a company like World, to undertake actions before the consummation of a transaction.
B. Scalability
No manual system has or will ever, prove fraud detection. However, AI can network process thousands of transactions simultaneously across various channels, thus becoming a truly applicable solution to organizations of any size.
C. Cutting Down Costs
Automated fraud detection reduces the human intervention required, which translates into greatly reduced operational costs most efficiently and accurately.
D. Enhanced Accuracy
AI will reduce false positives and negatives that wrongfully identify real fraudulent transactions. This will thus improve business continuity as well as the customer’s experience.
E. Customer Satisfaction And Trust
AI fraud detection is an avenue through which a company can build customer trust and goodwill without much friction by ensuring safe transactions and improving its users’ experience.
Challenges Of AI Fraud Detection
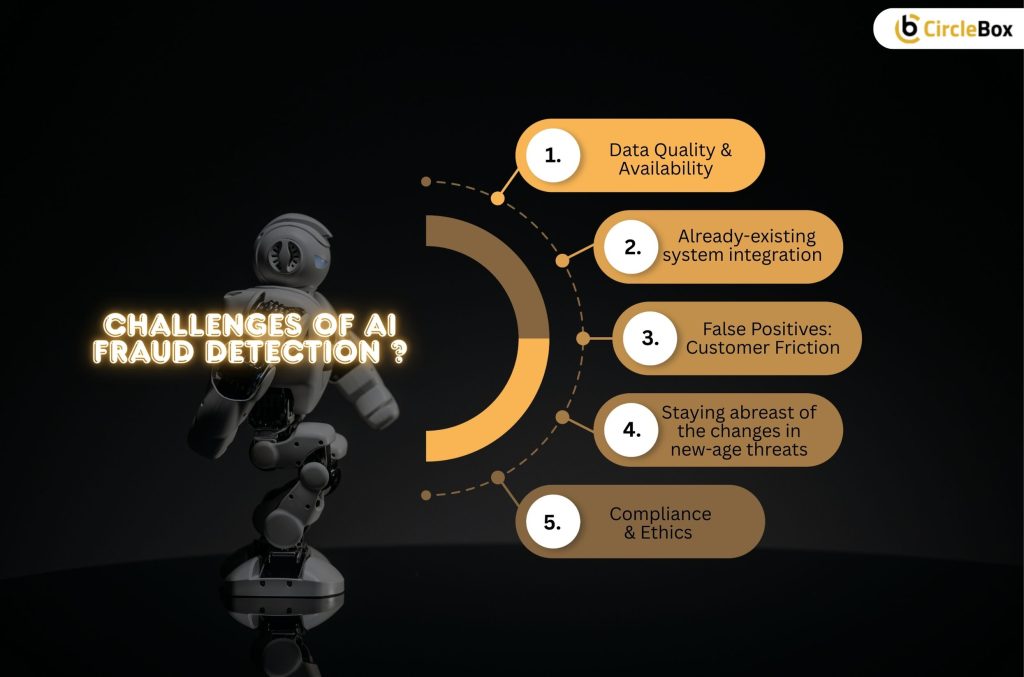
When it comes to AI fraud detection, there are a few challenges or problems you need to understand to find a solution. So here are a few problems of AI fraud detection.
1. Data Quality And Availability
For fraud detection, AI models need high-quality, diverse, and current data. Incomplete, biased, or out-of-date data can markedly shape the accuracy of any predictions made by an AI model.
2. Already-Existing System Integration
Companies often operate legacy systems prima facie incompatible with state-of-the-art AI-based model systems for fraud detection. It requires heavy investments in IT infrastructure and software upgrades concerning the extent of these integrations.
3. False Positives: Customer Friction
Aggressive fraud detection models often regard genuine transactions as abnormal, resulting in customer discomfort and financial losses. The contradiction is finding a fine balance between user experience and fraud prevention.
4. Staying Abreast Of The Changes In New-Age Threats
The criminals are also devising ways to get around AI-based detection. AI model updates and their security infrastructures should be regularly performed so that the organization can counter new threats.
5. Compliance And Ethics
Strong data protection legislation requires business organizations to adhere to ethical conduct when using AI-based fraud detection software.
Building An AI Fraud Detection Strategy

The instilling of the below-listed best practices is imperative for the successful implementation of a modern-day AI fraud detection measure:
A. Constitute A Cross-functional Fraud Management Team
With a dedicated team comprising data scientists, cybersecurity professionals, compliance officers, and fraud examiners, end-to-end solutions can be raised toward mitigating fraud by a fraud management team.
B. Continuous Monitoring And Updates
Continuous monitoring and development are essential for all AI systems in light of ever-changing fraud schemes. This also ensures continuing periodic audits and updates to improve the detection quality.
C. Building A Holistic Fraud Detection Strategy
This has implications for an organization’s existing fraud management scheme, namely that fraud detection goals, risk assessment methods, and incident response strategies should be clearly defined.
D. Fund For Best Tools
The chosen AI fraud detection solution must satisfy all business needs. Invest in AI platforms that ensure deep analytics, real-time operations, and seamless integration for future effectiveness.
E. Ethical Data Handling Practices
Organizations can apply ethical AI methods to obey regulations like GDPR and CCPA and keep customer data out of their reach.
F. Attack Simulation And Stress Testing
Simulations of fraud cases by penetration testing enable the organization to evaluate the strength of its AI fraud detection programs while highlighting their weaknesses.
G. Instill A Security Culture
Training on fraud threat awareness, cybersecurity best practices, and fraud detection processes nurtures a security-aware workforce and growing organizational fraud protection.
Final Thoughts
Artificial intelligence-based detection systems are changing the paradigm for organizations against financial fraud and cyber attacks.
Machine learning and real-time analysis provide a pull to detection rates and RM blocking of fraud; however, several integration hurdles and regulatory compliance must be crossed before one can envisage a full-blown AI-ready-oriented fraud detection.
A clear structure concerning AI fraud detection entails organizations being ahead of the fraudster with no traceability, garnering client trust, and complying with regulations.
Considering fast-paced advancements in AI, organizations will have to keep enhancing their fraud prevention systems, committing resources to innovate, and boosting security awareness concerning monetary transactions or any sensitive information.
Read Also: